Why Traditional Heatmaps are Failing Retailers and What’s Next
For decades, retailers have relied on heatmaps, or 2D visualizations of in-store traffic, as a tool to understand how shoppers move through the space, where they spend their time, and what products they see. From this information, retailers and CPGs have hoped to optimize their retail spaces and offerings to encourage shoppers to spend more time and money in stores. There’s just one problem: traditional methods of creating heatmaps are resulting in flawed and incomplete data.
Heatmaps have long been a go-to tool for retailers, but they are an inaccurate way of understanding engagement. Retailers are not getting the full picture of what is happening in their stores because current heatmaps, like a long exposure photograph, show concentrations of activity. Unfortunately, visualizing in-store activity this way conflates shopper volume and shopper dwell time, and cannot differentiate shoppers from store employees. What these businesses need to know is where customers go and exactly what they do when they get there – that’s where Standard AI comes in.
With Standard AI’s VISION Analytics Platform, accurate heatmaps are just one tool unlocking a vast amount of data available to retailers for the first time. VISION’s advanced approach to shopper modeling represents each facet of shopper behavior in a way that’s impossible with traditional heatmapping. For instance, our machine learning models and tracking system can easily decipher between shopper and employee movement in stores with a high degree of accuracy.
How Traditional Heatmaps are Misleading Retailers
Heatmaps today attempt to show retailers how shoppers are moving through their stores, but several fundamental flaws in data collection and processing mislead retailers into thinking they are looking at shopper movements when they are not.
Most systems used to create heatmaps don’t understand the relationship between different camera views in the store and, more importantly, do not have the ability to correlate the shopper seen in one camera view with that same shopper in a different view. Because most stores are large and complex enough that multiple cameras are required to see shoppers throughout the shopping floor, this is an immediate problem.
>
In multi-camera setups in which there is no correlation between shoppers, it is impossible to build a heatmap based on shopper pathing, or the actual path each shopper took through the store.
Rather, these systems measure human dwell time in a specific portion of the camera view so that each view can be independently analyzed and combined to create the heatmap.
Once you understand how these maps are made, the flaws become apparent. A single shopper standing in one spot for a long time can produce the same measure of “traffic” as multiple shoppers moving more quickly through an area.
This problem is only compounded when you consider these same systems cannot differentiate between actual shoppers and store employees.
Imagine, as a retailer, looking at a heatmap and interpreting a particular end cap as highly trafficked and therefore as a good spot for promoting new products, when in fact, what you were really seeing was your own employee restocking shelves or conducting routine tasks.
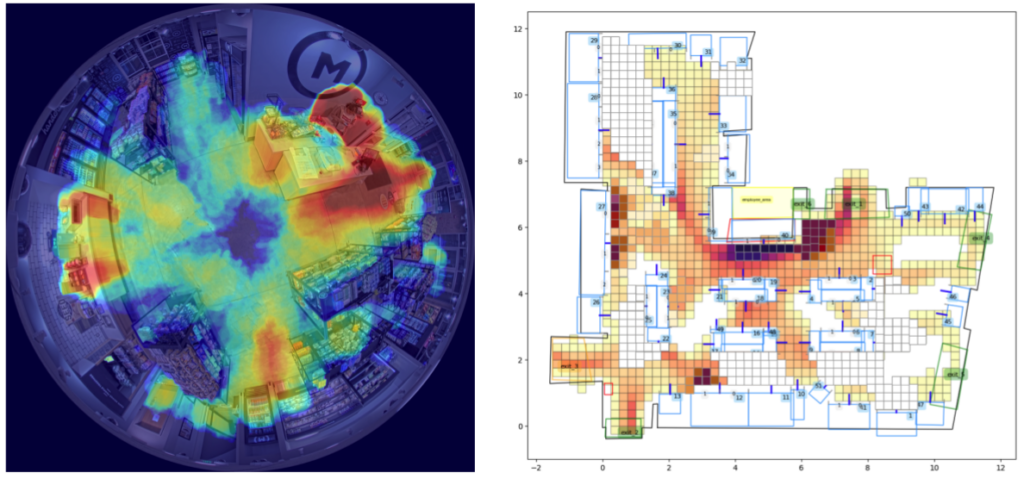
Figure 1 shows an example of a heatmap created using this long exposure technique. On the left, we see the heatmap overlaid on the camera view itself and on the right, we can see that same data flattened to a 2D map grid over the store layout. Based on the data above, you might reasonably conclude that, beyond the checkout counter in the middle of the floorplan, traffic concentrates near the coffee stations (on the left of both images and the shelf sections nearest the bottom left exit.
The fundamental difference between previous approaches to creating heatmaps and Standard AI’s approach is that the advanced heatmaps created by our VISION platform are precise visual representations of each shopper’s path through the store. With these heatmaps, retailers and CPGs are empowered to start making informed, sound decisions, rather than engaging in guesswork, or worse, being misled by their tools.
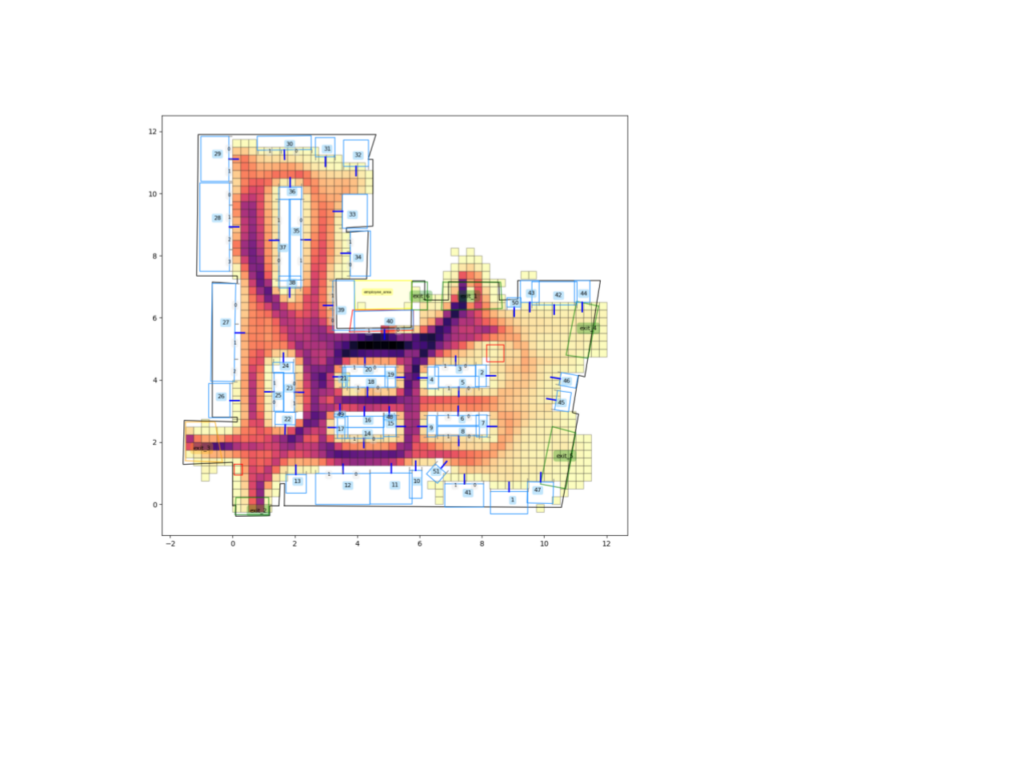
Figure 2 illustrates true traffic patterns based on Standard’s VISION platform. Rather than capturing exposure and conflating dwell time with traffic, we created the above map by counting actual traffic through each grid on the floor.
Immediately, we can draw two comparisons. First, because we create a 3D model of the entire store and eliminate blind spots in coverage, we can see a more complete traffic pattern in Figure 2 rather than a few areas of concentration within view of a single camera (as seen in Figure 1).
>
More importantly, we can see the concentration of traffic in Figure 1 around the coffee bar was just long dwell and not actual traffic. Shoppers at the coffee bar have to open and stir in milk and sugar, fiddle with the lid, and potentially microwave the coffee to heat it up. Store employees spend time straightening the coffee condiments and wiping down the counter.
The heatmap generated without a tracking approach hides this. While it’s useful for retailers to understand where shoppers spend their time in stores, that’s all a long exposure approach offers and even then, with an incomplete picture.
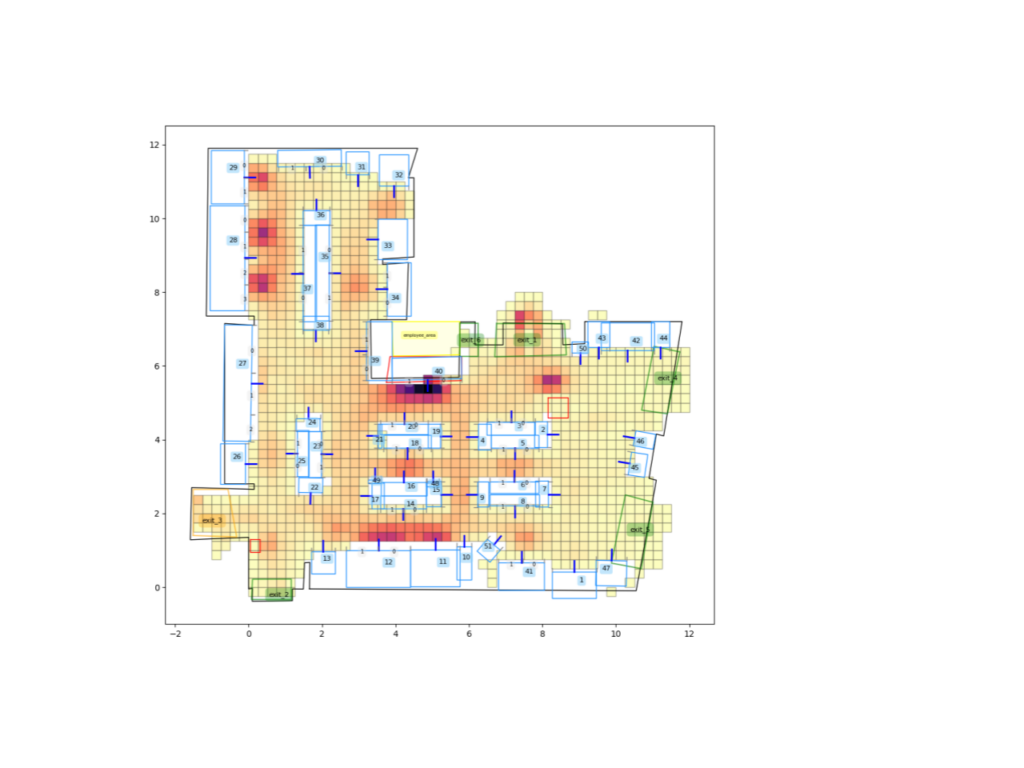
Taking this one step further, we can also count the frequency of stops within the space. Figure 3 illustrates the areas within the store in which most shoppers stopped, regardless of dwell time. Figure 3 shows us more distinctly that it’s really the dwell time of a few shoppers at the coffee bar skewing the numbers and more shoppers stop near the coolers in the top left and bottom middle of the floorplan.
Unlocking the Full Potential of Heatmaps with Advanced Computer Vision
When it comes to revolutionizing the retail experience for shoppers, retailers, and CPGs, what sets Standard AI apart is our computer vision technology. Using only overhead security cameras, we create sophisticated 3D models of every individual to anonymously track their movement. While other store traffic solutions struggle to monitor shoppers as they move from one camera to the next, our 3D spatial reconstruction maintains a seamless track of every shopper and employee – all while ensuring complete anonymity and safeguarding privacy, with no facial recognition involved.
To truly understand customer behavior at a micro level, simply tracking where people go and how long they stay barely scratches the surface of what is possible. For example, the time spent in transit through the store should not be given the same weight as the time spent contemplating products on the shelf. Not only can our VISION platform understand shopper pathing, but because we create 3D representations of each shopper moving through the store, we can measure subtle changes in a shopper’s velocity, orientation and gaze, allowing us a much richer model of shopper behavior including transiting, impression formation, and queueing. It’s crucial to dig deeper to truly understand who visits which areas of the store and what they do once they get there.
Another benefit of Standard’s VISION platform is that, while we create high-fidelity models of shoppers and their movements through a store, we do so in a way that preserves each shopper’s privacy. Standard’s algorithms have been built from the ground up to be privacy-first. We don’t use facial recognition or other sensitive biometrics to track shoppers and while we know where people are in a store, we never know who they are.
Our Vision for Retail Insights
Standard AI has the unique opportunity to leverage best-in-class computer vision in physical retail spaces. The richness of Standard AI’s data empowers retailers to take action on real-time insights, helping them improve operations, drive revenue, and boost return on investment. By taking existing camera footage and applying computer vision technology, retailers now have access to a plethora of meaningful insights, advanced heatmaps being one of them. With next-generation AI-powered heatmaps, retailers and CPGs will be more empowered to start making informed decisions and real impact on their bottom line.